Credit Rating Model Building: A Deep Dive for Finance Professionals
Meta Description: Master credit rating model building with this in-depth guide. Learn about model design, factor selection, handling industry cycles, and model validation—all backed by real-world examples and expert insights. Keywords: Credit Rating Model, Financial Modeling, Risk Assessment, Industry Cycles, Model Validation, Credit Risk
Hey there, future financial wizards! Are you ready to unlock the secrets of building a truly powerful credit rating model? Forget dry textbooks and theoretical jargon—we're diving headfirst into the real-world challenges and triumphs of creating a model that's not just accurate, but also insightful and actionable. This isn't your grandpappy's credit scoring system; we’re talking cutting-edge techniques, practical solutions, and a healthy dose of real-world experience from the trenches of financial modeling. We'll unravel the complexities of handling diverse industry characteristics, integrating operational data with financial metrics, and expertly navigating the ever-shifting sands of macroeconomic influences. Prepare to level up your financial analysis skills and build a robust credit rating model that will not only impress your boss but also empower your organization to make smarter, safer investment decisions. Let's face it, in today's volatile market, a rock-solid credit risk assessment model is a MUST-HAVE, and this comprehensive guide is your roadmap to success! This isn't just theory; we’re serving up actionable strategies, real-world examples, and practical tips to help you build a truly effective credit scoring system. Buckle up, because this journey is going to be epic!
Why Build a Credit Rating Model?
Let's be honest, in the whirlwind of today's financial markets, simply relying on gut feeling is a recipe for disaster. A robust credit rating model isn't just a nice-to-have; it's a lifeline for smart investing and risk management. Think of it as your financial compass, guiding you through the stormy seas of uncertainty. So, why bother building one? Here's why it’s a game-changer:
-
Unified Approval Standards: Say goodbye to inconsistent decisions and hello to standardized risk assessment. A single, well-defined model ensures everyone's on the same page, regardless of their department or personal biases. That means more consistent and predictable outcomes, leading to greater efficiency and reduced errors.
-
Sharper Risk Pricing & Investment Decisions: Move beyond simple "yes/no" credit assessments. A sophisticated rating system provides granular risk scores, enabling more precise risk-return trade-offs. This allows for the creation of optimized investment portfolios, maximizing returns while minimizing risk.
-
Enhanced Risk Monitoring & Early Warning Systems: Think of it as having a financial alarm system. The model sets thresholds, triggering alerts when risk scores dip below a certain level. This enables proactive risk management, allowing for timely interventions such as reducing exposure or demanding additional collateral before things go south.
-
Adaptability to Dynamic Markets & Policy Shifts: Financial landscapes are constantly changing. A well-designed model can quickly adapt to new data and market conditions, providing real-time risk assessments. This agility is crucial for staying ahead of the curve in a competitive environment.
-
Knowledge Capitalization & Experience Transmission: Formalizing expertise into a model transforms individual knowledge into a valuable organizational asset. It reduces reliance on specific individuals, facilitating knowledge sharing and minimizing disruptions caused by staff turnover.
Industry-Specific Model Exposure: Defining the Scope
This is where things get really interesting. One size doesn’t fit all, especially in the world of credit risk. Different industries have vastly different financial characteristics, and ignoring this reality can lead to inaccurate and unreliable results. We need to carefully define the scope of our model to ensure it's tailored to the specific characteristics of each industry.
For example, we might initially categorize borrowers into broad groups like industrial firms, municipal entities (munis), and financial institutions. These groups have fundamentally different risk drivers. Munis rely heavily on government support, industrial firms operate in a more market-driven environment with documented defaults, and financial institutions have unique operational models and accounting structures. Treating them all the same is a recipe for disaster.
At DM, using a sophisticated methodology, we analyzed thousands of industrial borrowers, discovering that operating models heavily influence financial performance. Default causes varied significantly across sectors. Building a single model for all industries was impossible; it would only yield indicators effective for the majority, missing crucial sector-specific signals. Moreover, not all sectors had sufficient default data for individual modeling.
Our solution? We meticulously reviewed historical default cases, identifying key characteristics. Using clustering algorithms, we grouped 37 industries into two distinct exposure categories: manufacturing and non-manufacturing. This ensured sufficient bad-debt samples within each group for improved model accuracy and applicability of selected indicators.
Quantifying Financial Factor Selection: Linking Financials to Defaults
Building a financial model involves considering numerous dimensions: debt repayment capacity, growth potential, funding capabilities, size, investment prowess, cash flow, operational efficiency, profitability, capital structure, and asset quality. At DM, our initial checklist included over 240 financial metrics!
Before selecting effective indicators, we grouped similar metrics to balance diversity and reduce the risk of over-reliance on any single variable. Data cleaning is paramount. For average metrics, missing data was replaced with the average of available data; for growth rates, we used absolute values to avoid negative numbers; and for ratios, we used minimum or maximum values to handle missing denominators. This attention to detail ensures model robustness.
We employed several methods for quantitative factor selection:
-
Missing Rate: Eliminating variables with over 40% missing data ensured data viability.
-
Stability: We used the Population Stability Index (PSI). Indicators with PSI > 0.25 were considered unstable and removed.
-
Default Discrimination: We used the Area Under the ROC Curve (AUC) and Information Value (IV) to assess a variable’s ability to distinguish between defaulting and non-defaulting borrowers. Variables with low AUC or IV were excluded.
-
Correlation: To avoid multicollinearity, we checked for high correlations between metrics (above 0.85), keeping only the most discriminating variable.
Raw data often contains noise and outliers. Instead of using raw data directly, we transformed it into continuous default rates, ensuring a unique default rate for each metric value. This process involves:
- Dividing the data into quantiles (e.g., 20 quantiles).
- Calculating the default rate for each quantile.
- Applying local regression for smoothing.
- Enforcing monotonicity (default rate increasing or decreasing monotonically with the variable's value).
- Using a cumulative density function (CDF) to map each raw value to its corresponding default rate.
This approach improved model stability and predictive power while preserving interpretability.
Blending Operational Performance and Financial Health
Traditional credit models often focus solely on financial statements due to data availability and simplicity. However, financial data lags, and not all operational performance is reflected in financials. Therefore, we integrated operational and financial data. Our framework combines qualitative assessments with quantitative modelling.
For operational assessment, we collected and organized over 500 operational indicators across 37 industries. Using univariate analysis, we selected effective and model-suitable indicators, creating industry-specific scoring cards. These cards incorporate expert judgment for accurate and practical results. Financial assessment used a regression model with default as the dependent variable, identifying key predictors of default risk.
We integrated operational and financial results using a generalized linear logit model. This model ensures that combining the two provides more predictive power than either alone. The model's output is a continuous variable, facilitating credit risk grading. Parameter selection combines expert input and quantitative testing.
Incorporating Macro and Micro Risk
After integrating operational and financial assessments, we adjusted ratings across industries to ensure comparability. This accounts for differing industry cycles and business conditions. Our analysis considers industry revenue and profitability cycles, entry barriers, competition, stability, and supply chain structure. We developed a meso-level business cycle index (DM) for each industry, using indicators closely related to industry revenue and net profit to forecast business conditions. These indicators are weighted based on their correlation with market experience.
The business cycle index adjusts the mixed model's parameters (intercept and slope) to correct the distribution of expected default rates without altering the relative rankings of borrowers within each industry. This involves:
- Setting target rating distributions for each industry based on the business cycle index.
- Identifying low and high-risk rating anchors based on expert opinions.
- Optimizing model parameters to match the target distributions.
Validating Model Effectiveness
Model validation is crucial. We evaluate a model's accuracy, ensuring reliable assessment results. Key aspects include:
-
Discrimination: This measures the model's ability to rank borrowers correctly. We analyze default and non-default rating distributions, using metrics like KS statistics, AUC and AR to assess performance. Low discrimination in specific industries may indicate the need for adjustments.
-
Stability: This assesses the model's generalization ability to new samples, using the PSI to compare rating distributions between training and validation data. Cross-cyclical validation strengthens the model's robustness.
-
Predictive Power: We assess the model's ability to predict defaults by analyzing the ratings of defaulted borrowers in the 1-2 years before default and creating transition matrices to assess the probability of default based on rating levels.
Frequently Asked Questions (FAQs)
Q1: What types of data are essential for building an effective credit rating model?
A1: The most crucial data includes financial statements (balance sheets, income statements, cash flow statements), operational data (sales, production, etc.), macroeconomic indicators (GDP growth, interest rates), and any industry-specific data relevant to the sector. High-quality data is paramount!
Q2: How do you handle missing data in the model development process?
A2: Missing data is a common challenge. We use various imputation techniques, such as mean imputation, median imputation, or more sophisticated methods like k-nearest neighbors (KNN) or multiple imputation, depending on the nature of the data and the extent of missingness. The choice of method depends on the characteristics of the data.
Q3: What are the key limitations of using only financial data in credit risk assessment?
A3: Financial data is inherently backward-looking and can lag behind changes in a company's operational performance. Operational factors often provide early warning signals of potential financial distress. Therefore, using solely financial data might miss crucial early warning signs.
Q4: How do you ensure the model remains relevant over time?
A4: Regular monitoring and updating are critical. The model's performance must be regularly reviewed using out-of-sample data, and the model's parameters recalibrated as needed to reflect changes in the market and economic conditions. This ensures its continued accuracy and relevance.
Q5: What are the ethical considerations in using credit rating models?
A5: Fairness, transparency, and accountability are paramount. The model should not discriminate against protected groups, and its methodology should be clearly documented and understandable. Regular audits and bias checks are crucial to ensure responsible and ethical use.
Q6: How can I improve the explainability and interpretability of my credit rating model?
A6: Use simpler models when possible (e.g., linear models, decision trees), employ feature importance analysis to identify the most influential variables, and create visualizations to understand the model's predictions and decision-making process. Documenting the model's development and limitations enhances transparency.
Conclusion
Building a robust credit rating model is a challenging but rewarding endeavor. It requires a deep understanding of finance, statistics, and programming, as well as a keen eye for detail and a commitment to continuous improvement. By combining sound theoretical frameworks with practical, real-world experience, you can create a model that provides valuable insights into credit risk, empowers informed decision-making, and ultimately contributes to the financial health and stability of your organization. Remember, this is an ongoing process; continuous monitoring, refinement, and adaptation are key to maintaining its effectiveness and relevance in the ever-evolving world of finance. So keep learning, keep experimenting, and keep building! Your financial future depends on it!
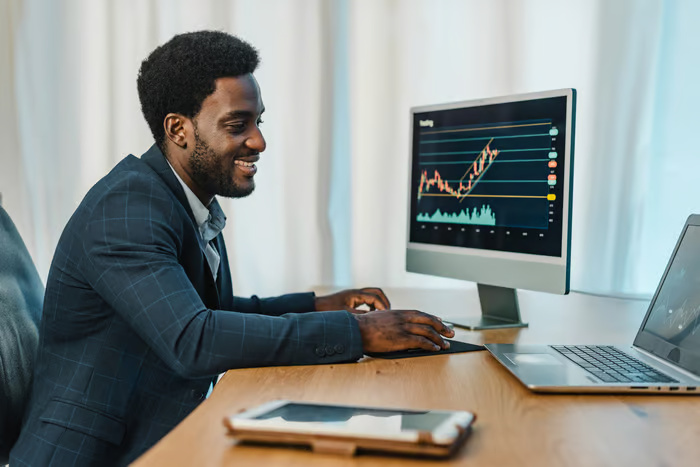